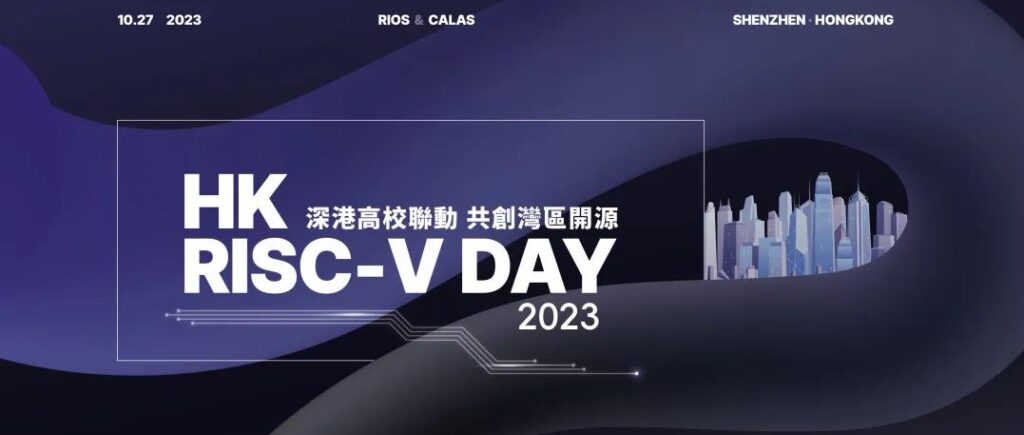
RIOS&CALAS HK RISC-V Day 2023
Since its establishment, the RISC-V International Open Source (RIOS) Laboratory has garnered widespread attention and support from various sectors of society. In order to further promote and enhance the development of the global RISC-V open-source technology ecosystem, RIOS, in collaboration with the CityU Architecture Lab for Arithmetic and Security (CALAS) of City University of Hong Kong, co-hosted the “RIOS & CALAS HK RISC-V Day 2023” on October 27-28, 2023.
The visit to Hong Kong aimed to deepen understanding of the academic and innovation ecosystem in Hong Kong, strengthen exchanges and cooperation between academia in Hong Kong and mainland China, promote academic research and knowledge sharing, and facilitate collaboration among domestic and international chip and computer industry leaders. Together, they explored the current development status and future opportunities of RISC-V in the Guangdong-Hong Kong-Macao Greater Bay Area.
On one hand, the laboratory showcased recent research achievements to industry experts and scholars; on the other hand, the laboratory invited frontline experts from internationally renowned universities and supercomputing centers to deliver exciting cutting-edge academic presentations on the new computing architecture revolution driven by RISC-V and core technologies of open-source chips. This initiative aimed to provide students with a more comprehensive understanding of the international academic environment, enhance their cross-cultural communication skills and professional capabilities, and lay a solid foundation for their future development on international platforms.
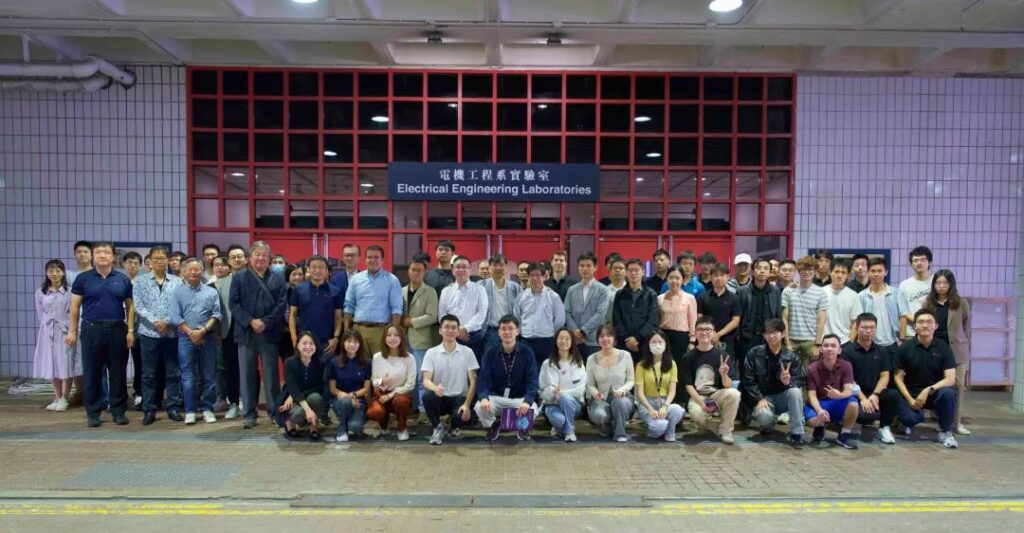
The Highlights of the Event
Cutting-edge Technology Presentations: The RIOS Laboratory showcased its advancements in chip design, large language models, AI chip engineering, low-level computer compilation, vector extension instruction set testing and verification, and Open EDA/PDK to renowned experts and scholars from around the world.
Student Achievements: Notable achievements by students were highlighted, including winning first place in international semiconductor design competitions and receiving awards for designing the world’s first AI-designed RISC-V CPU in the “AI-generated Open Source Chip Challenge.”
Special Presentations by Experts: World-class experts in relevant fields delivered specialized presentations, sharing insights into research trends and the latest developments in international advanced semiconductor initiatives, including open-source chip design and high-efficiency supercomputing architectures based on RISC-V and AI.
Personalized Guidance: Senior experts provided face-to-face, one-on-one guidance to students on their research topics and future development, fostering open and engaging discussions and providing invaluable mentorship.
Opportunities for Students: The event provided students with a rare opportunity to gain insights into the latest industry technologies, understand the newest innovations and technological trends in the industry, and maintain their research capabilities at the forefront of relevant disciplines.
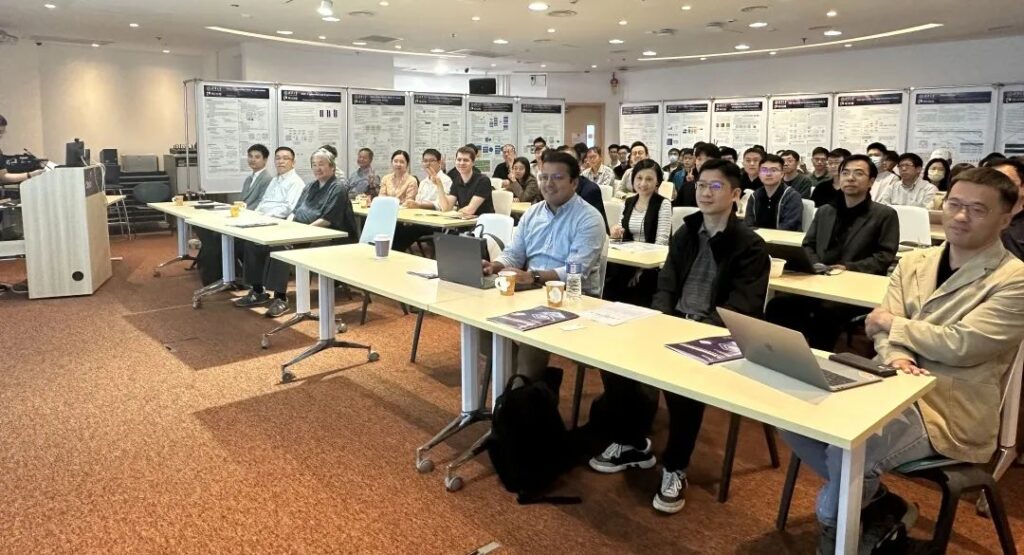
The RIOS Laboratory organized a trip for students to visit the Hong Kong Science Park, where they had the opportunity to tour multiple international research laboratories. This experience allowed them to gain firsthand knowledge of the forefront of innovation and technological development in the Guangdong-Hong Kong-Macao Greater Bay Area, as well as insights into the ecosystem and industrial prospects. The students found the experience to be highly enriching and gained valuable insights into the region’s innovation and technology landscape.
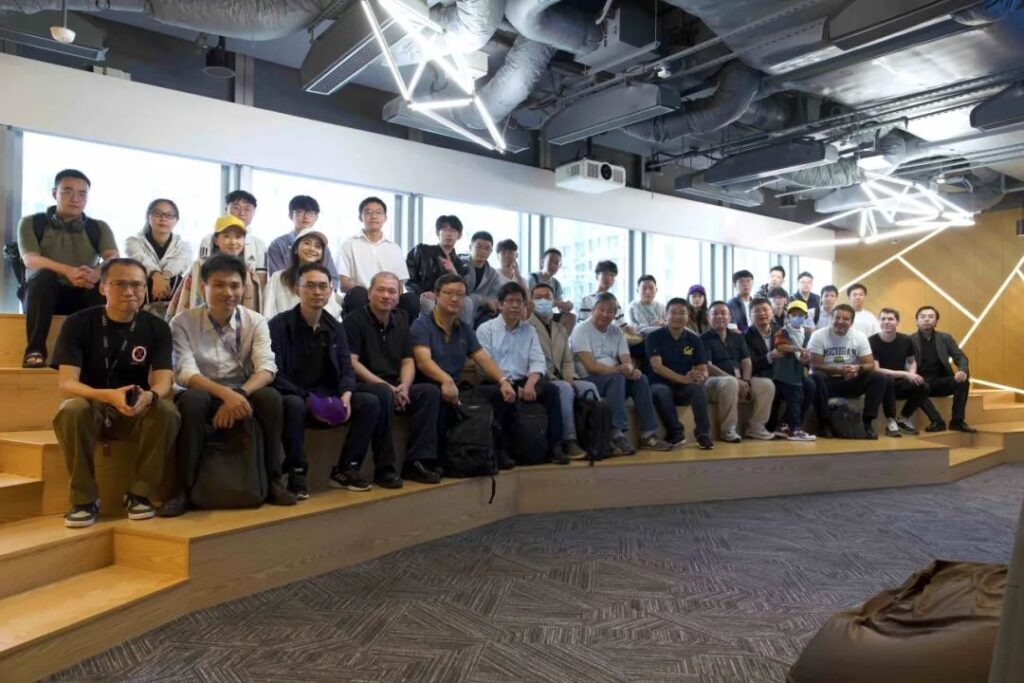
Original link: https://https://mp.weixin.qq.com/s/vYvrZ31iqHmF7URfEm8DYA